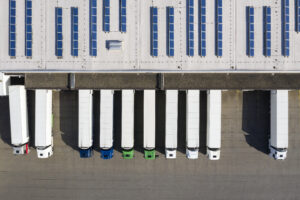
Brain Hub
Including white papers, client case studies, BRAIN UP and blog articles, here you will find all the useful resources to follow trends related to decarbonization and simplification of logistics planning.
Home / Ressources /
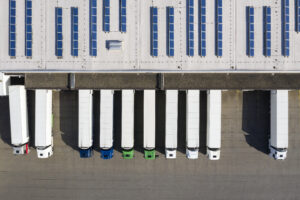
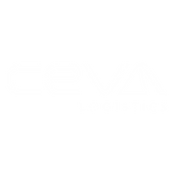
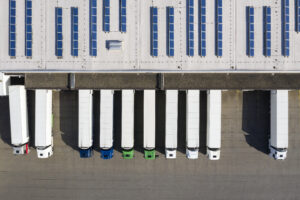
Ceva Facilitates the Design of its Routes with DCbrain
Read more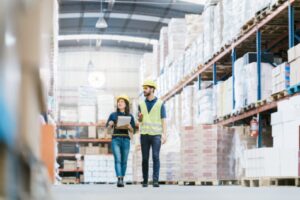
Lidl Enhances its Transportation Plans with DCbrain
Read more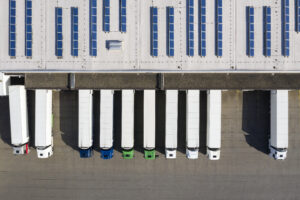
Stef Optimizes its Transportation and CO2 Emissions with DCbrain
Read more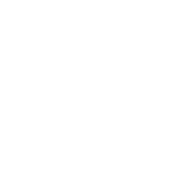
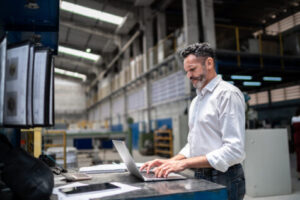
Daher Improves its Operational Performance with DCbrain
Read more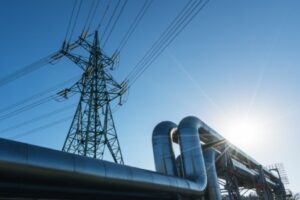